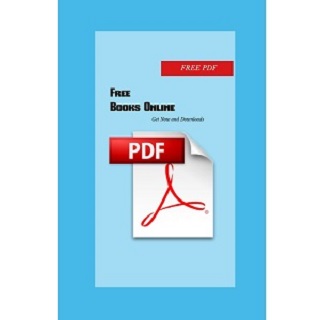
▶▶ Read Computer Vision: Models, Learning, and Inference Books


Detail books :
Author :
Date : 2012-06-18
Page :
Rating : 4.5
Reviews : 26
Category : Book

Reads or Downloads Computer Vision: Models, Learning, and Inference Now
1107011795
Computer Vision Models Learning and Inference ~ Simon Prince’s wonderful book presents a principled modelbased approach to computer vision that unifies disparate algorithms approaches and topics under the guiding principles of probabilistic models learning and efficient inference algorithms
Computer vision models learning and inference ~ Computer vision models learning and inference ©2011 Simon Prince Pinhole camera model is a nonlinear function that takes points in 3D world and finds where they map to in image Parameterized by intrinsic and extrinsic matrices Difficult to estimate intrinsicextrinsicdepth because nonlinear
06 Learning And Inference BobEdits ~ Computer vision models learning and inference ©2011 Simon Prince 34 In this example both generative and discriminative models lead to the same posterior normal distribution pwx if MLE is used to estimate model parameters This is due to both x and w
Computer Vision Models Learning and Inference 2019 ~ This class provides an introduction to three key components in computer vision 1 models 2 learning 3 inference While most of our focus in all these three components is on machinelearning approaches our scope is broader and includes topics from other branches of mathematics statistics computer science and electrical engineering A
Computer Vision Models Learning and Inference ~ This modern treatment of computer vision focuses on learning and inference in probabilistic models as a unifying theme It shows how to use training data to learn the relationships between the observed image data and the aspects of the world that we wish to estimate such as the 3D structure or the object class and how to exploit these relationships to make new inferences about the world from new image data
A Gentle Introduction to Computer Vision ~ — Page 83 Computer Vision Models Learning and Inference 2012 It is a multidisciplinary field that could broadly be called a subfield of artificial intelligence and machine learning which may involve the use of specialized methods and make use of general learning algorithms
Computer Vision Models Learning and Inference PDF ~ Computer Vision Models Learning and Inference This modern treatment of computer vision focuses on learning and inference in probabilistic models as a unifying theme It shows how to use training data to learn the relationships between the observed image data and the aspects of the world that we wish to estimate
Computer Vision Models Learning and Inference ~ This modern treatment of computer vision focuses on learning and inference in probabilistic models as a unifying theme It shows how to use training data to learn the relationships between the observed image data and the aspects of the world that we wish to estimate such as the 3D structure or the object class and how to exploit these relationships to make new inferences about the world from new image data
8 Books for Getting Started With Computer Vision ~ This modern treatment of computer vision focuses on learning and inference in probabilistic models as a unifying theme It shows how to use training data to learn the relationships between the observed image data and the aspects of the world that we wish to estimate such as the 3D structure or the object class and how to exploit these relationships to make inferences about the world from new image data
GitHub jbhuang0604awesomecomputervision A curated ~ Computer Vision Computer Vision Models Learning and Inference Simon J D Prince 2012 Computer Vision Theory and Application Rick Szeliski 2010 Computer Vision A Modern Approach 2nd edition David Forsyth and Jean Ponce 2011 Multiple View Geometry in Computer Vision Richard Hartley and Andrew Zisserman 2004
0 Comments:
Post a Comment